
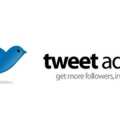
Online social media platforms have paved the way greatly into the lives of people and made person-to-person interaction faster by making the world more connected. We also prove the effectiveness of the proposed interaction and semantic consistency detection. Extensive experiments demonstrate that our framework outperforms competitive baselines on a comprehensive Twitter bot benchmark. Besides, BIC contains a semantic consistency detection module to learn semantic consistency information from tweets. Specifically, BIC contains a text propagation module, a graph propagation module to conduct bot detection respectively on text and graph structure, and a proven effective text-graph interactive module to make the two interact. In this paper, we propose a novel model named BIC that makes the text and graph modalities deeply interactive and detects tweet semantic inconsistency. However, hardly a method has made text and graph modality deeply fused and interacted to leverage both advantages and learn the relative importance of the two modalities. In addition, methods leveraging Twitter graph structure are recently emerging, showing great competitiveness. These novel bots are proposed to be characterized by semantic inconsistency. However, novel Twitter bots evade these detections by stealing genuine users' tweets and diluting malicious content with benign tweets. Existing text-based methods can deeply analyze user tweet content, achieving high performance. Twitter bot detection is an important and meaningful task. Our experimental evaluation demonstrates the efficacy of the proposed classification system. It uses the combination of features extracted from an unknown user to determine the likelihood of being a human, bot, or cyborg. Based on the measurement results, we propose a classification system that includes the following four parts: 1) an entropy-based component, 2) a spam detection component, 3) an account properties component, and 4) a decision maker. We observe the difference among human, bot, and cyborg in terms of tweeting behavior, tweet content, and account properties. We first conduct a set of large-scale measurements with a collection of over 500,000 accounts. To assist human users in identifying who they are interacting with, this paper focuses on the classification of human, bot, and cyborg accounts on Twitter. More interestingly, in the middle between human and bot, there has emerged cyborg referred to either bot-assisted human or human-assisted bot.

Legitimate bots generate a large amount of benign tweets delivering news and updating feeds, while malicious bots spread spam or malicious contents. The popularity and open structure of Twitter have attracted a large number of automated programs, known as bots, which appear to be a double-edged sword to Twitter. Users communicate with each other by publishing text-based posts. Twitter is a new web application playing dual roles of online social networking and microblogging.
